5 & 6 Neural Networks and Back Propagation
About 2238 wordsAbout 7 min
notescomputer-vision
2024-12-21
Summary:
Part 1: Neural Networks, Activation Functions, Biological Neurons, Space Warping, Universal Approximation, Convergence of Neural Networks
Part 2: Backpropagation, Computational graphs, Modular implementation, automatic differentiation, Higher-order derivatives
@Credits: EECS 498.007 | Video Lecture: UM-CV
Personal work for the assignments of the course: github repo.
Notice on Usage and Attribution
These are personal class notes based on the University of Michigan EECS 498.008 / 598.008 course. They are intended solely for personal learning and academic discussion, with no commercial use.
For detailed information, please refer to the complete notice at the end of this document
Neural Networks
Linear classifiers are limited to their linear decision boundaries. Neural networks are more flexible and can learn more complex decision boundaries.
Feature extraction
Color histograms, HOG, SIFT, etc. (2000-2010)
- Color histograms - Statistical Method
- HOG: Histogram of Oriented Gradients
- Compute edge direction/strength at each pixel
- Bag of Words(Data-Driven) - Clustering
- Extract random patches from images &Cluster patches into "codebook" of visual words
- Encode each image as histogram of visual words
- Concatenate features together - Different methods can capture different features of the image
2011 ImageNet Winner: A complicated feature extractor
SIFT: Scale-Invariant Feature Transform 128-D vector
color: 96-D vector
Reduced to 64-D with PCA
FV extraction and compression
One-vs-all SVM learning with SGD
Late fusion of SIFT and color systems
Problem: These complicated systems are not designed to directly minimize the classification error!
AlexNet: Deep Learning (2012)
Deep Neural Networks (DNNs) are end-to-end trainable!
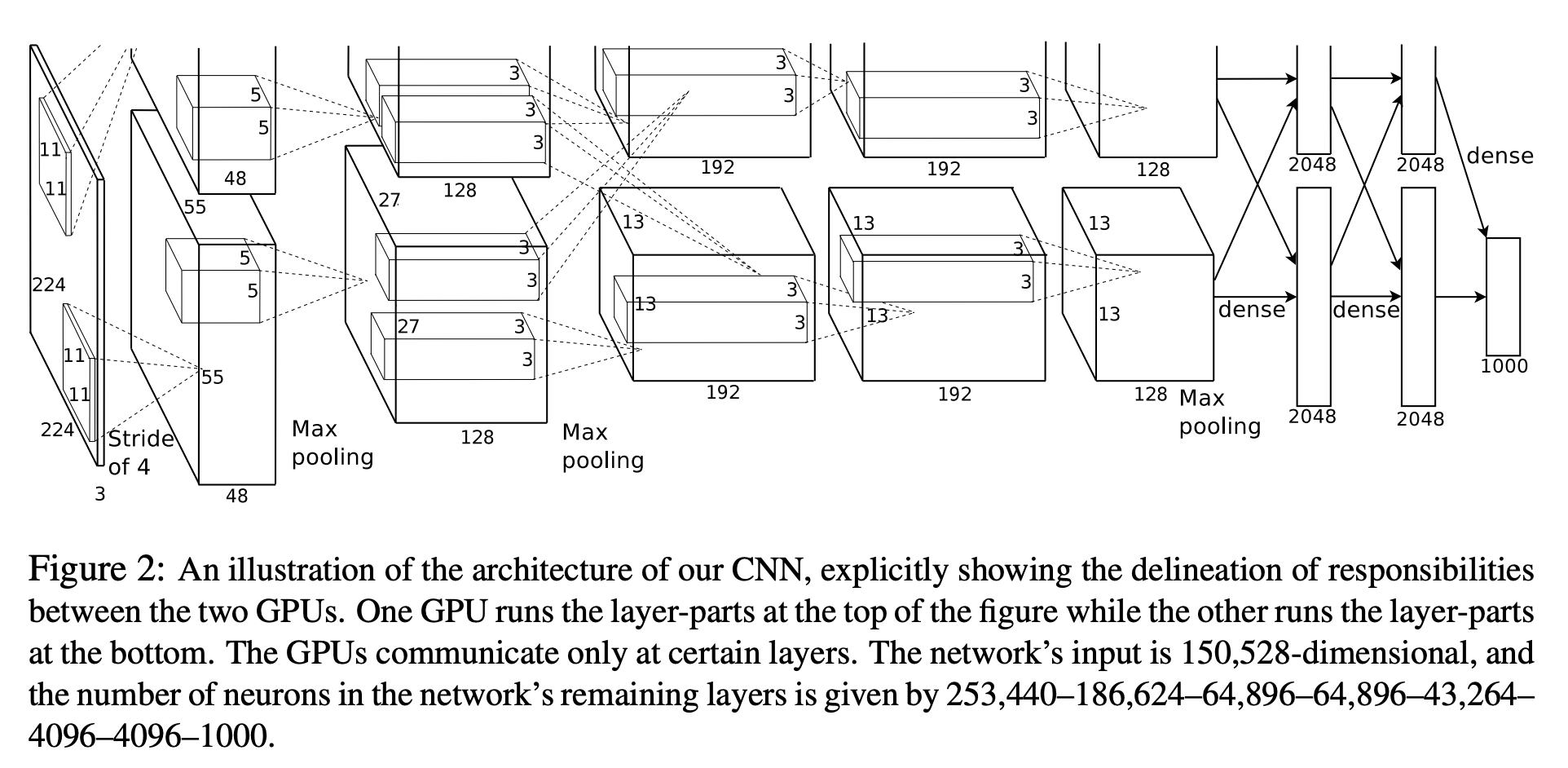
Fig: AlexNet
Neural Networks
(Before) Linear score function: f(x,W)=Wx
(Now) 2-layer Neural Network: f(x,W)=W2max(0,W1x)
where x∈RD,W1∈RH×D, W2∈RC×H
or 3-layer Neural Network
f(x,W)=W3max(0,W2max(0,W1x))
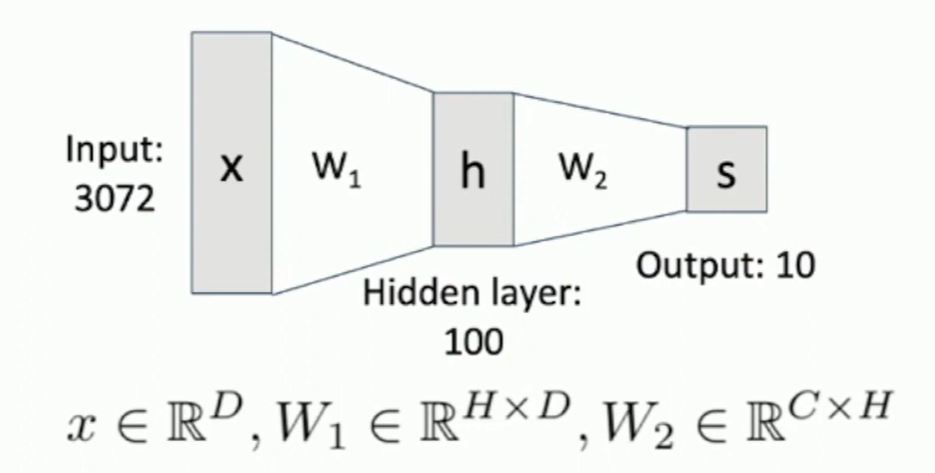
Fig: Data Flow
Deep Neural Networks
Each output is affected by each input: Fully connected network (or MLP - Multi-Layer Perceptron), and templates (each row of the matrix W) are combined layerwise.
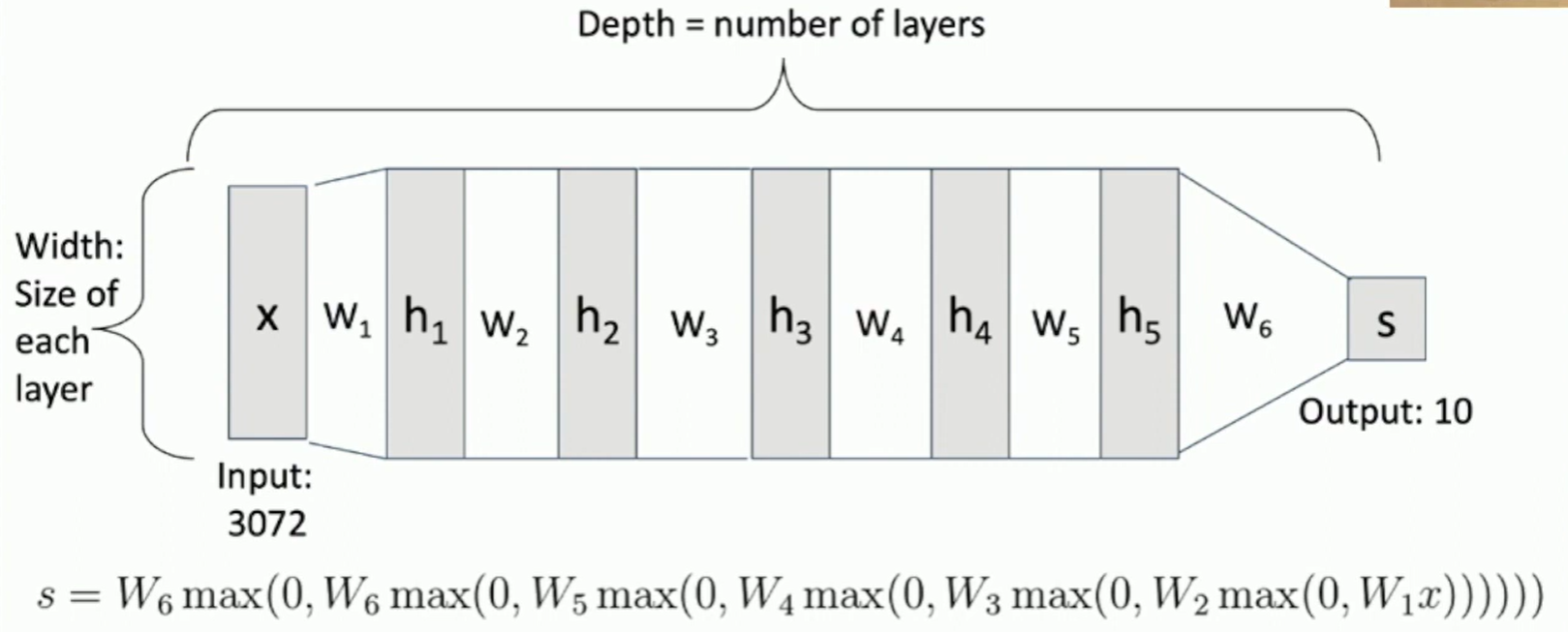
Fig: DNN
Activation Functions
Add non-linearity to the network. Without non-linearity, the network would be equivalent to a single layer.
The most widely used: ReLU (Rectified Linear Unit)
ReLu(x)=max(0,x)
Sigmoid
σ(x)=1+e−x1
Tanh
tanh(x)=ex+e−xex−e−x
Leaky ReLU
Leaky ReLU(x)=max(0.01x,x)
Maxout
Maxout(x)=max(w1Tx+b1,w2Tx+b2)
ELU
ELU(x)={xα(ex−1)if x>0if x≤0
Neural Network in < 20 lines!
import numpy as np
from numpy.random import randn
N, Din, H, Dout, = 64, 1000, 100, 10
x, y = randn(N, Din), randn(N, Dout)
w1, w2 = randn(Din, H), randn(H, Dout)
learning_rate = 1e-6
for t in range(10000):
h = 1/(1 + np.exp(-x.dot(w1)))
y_pred = h.dot(w2)
loss = np.square(y_pred - y).sum()
grad_y_pred = 2.0 * (y_pred - y)
grad_w2 = h.T.dot(grad_y_pred)
grad_h = grad_y_pred.dot(w2.T)
grad_w1 = x.T.dot(grad_h * h * (1 - h))
w1 -= learning_rate * grad_w1
w2 -= learning_rate * grad_w2
Analogy: Biological Neurons
Our brains are made of neurons. Impulses are carried away from cell body. Dendrites receive impulses from other neurons. Synapses are connections between neurons.
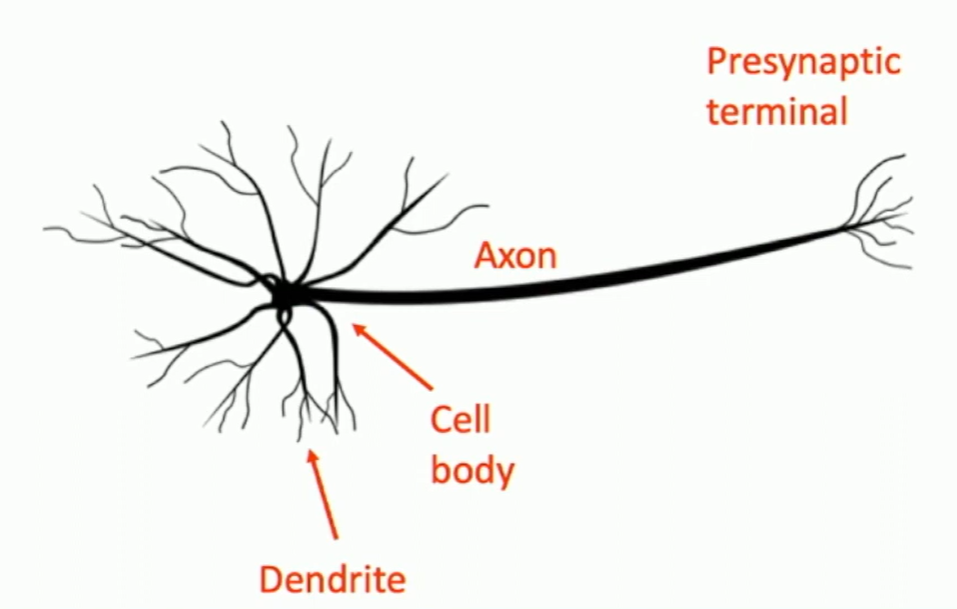
Fig: Biological Neurons
Differences between biological and artificial neurons
- Biological neurons have complex connectivity patterns
- Inside a neuron, signals are computed by complex processes
- Artificial neurons are organized into regular layers for computational efficiency
Fun fact: But neural networks with random connectivity can still learn!
Space Warping
A linear layer cannot improve the representation power of the classifier, but with a non-linear transform in between, the classifier can learn more complex decision boundaries.
Explanation: Space Warping
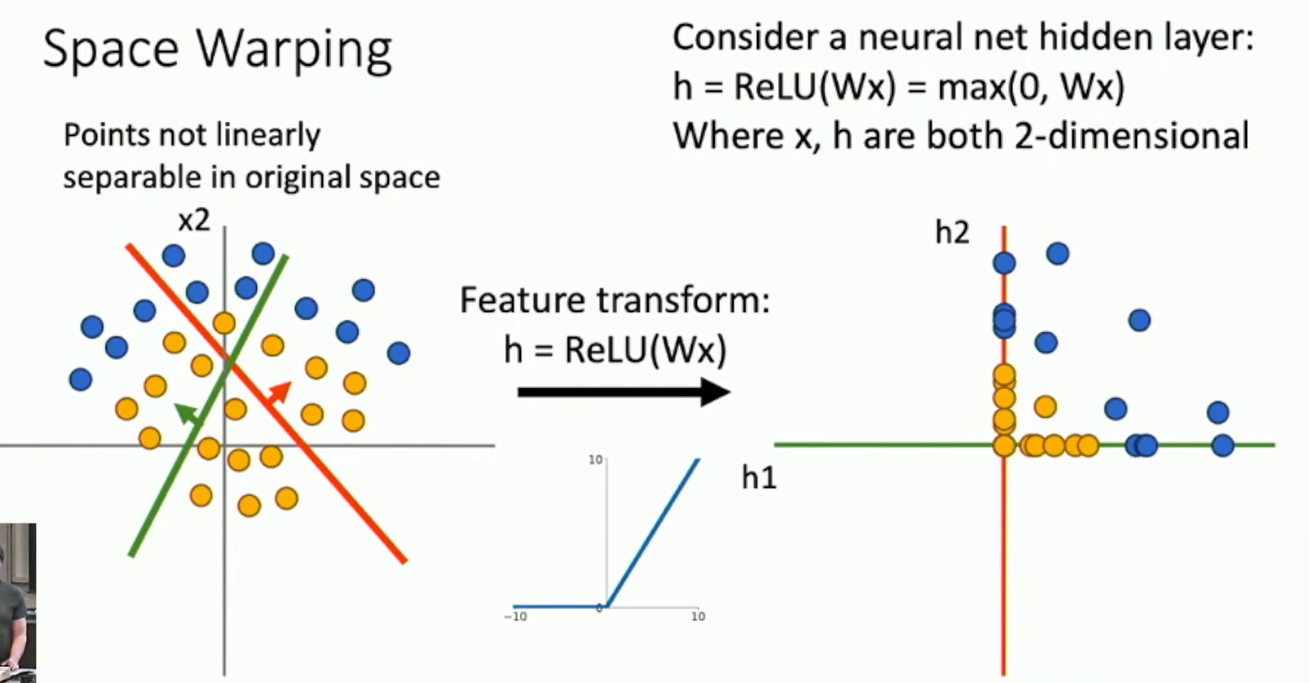
Fig: Space Warping
Points can be linearly separable in a higher-dimensional space.
Technique: Don't regularize with size; instead use stronger L2.
Universal Approximation
A neural network with a single hidden layer can approximate any continuous function to arbitrary accuracy (uniformly convergent), on compacts of Rn.
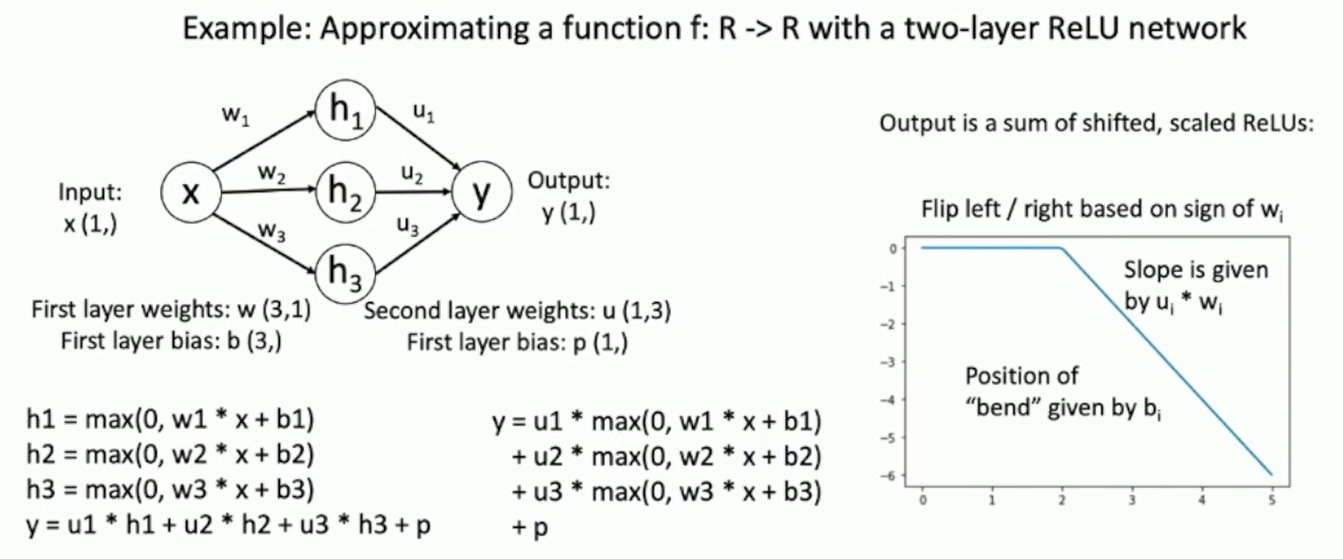
Fig: Universal Approximation
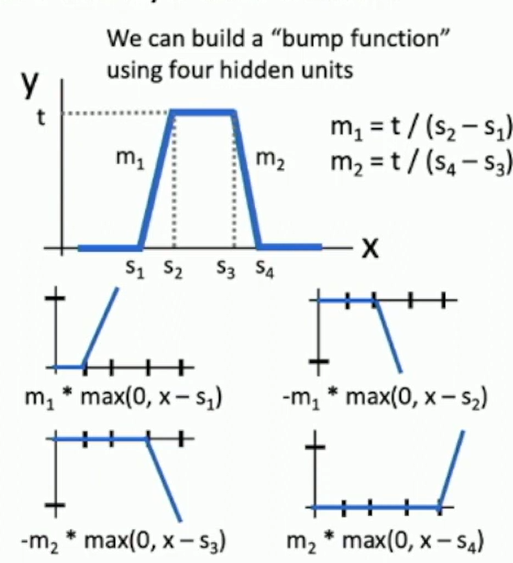
Fig: Bump function as basis
See Nielsen's Neural Networks and Deep Learning for more details.
Reality check: Networks don't really learn bumps!
But the theorem does not tell us how to find the right weights, given that f is unknown a priori.
Convergence of Neural Networks
Definition: (Convexity) A function f:RD→R is convex if for all x,y∈RD and t∈[0,1], we have
f(tx+(1−t)y)≤tf(x)+(1−t)f(y)
Theorem: A single layer neural network with ReLU activation is a convex function of its weights.
No such guarantee for non-linear networks.
Neural net losses sometimes look convex-ish.
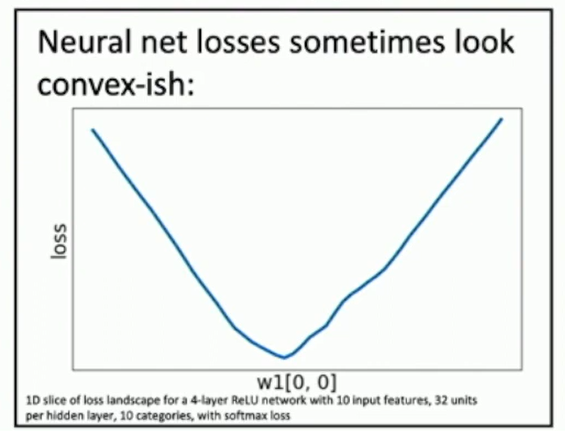
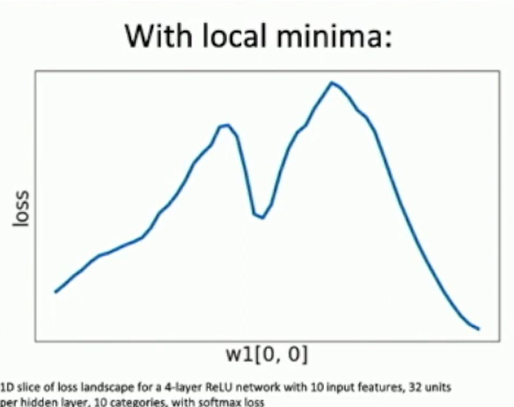
Fig: loss surfaces
Open question: theoretical properties of the optimization landscape of neural networks.
Summary of lecture 5
- Feature transform
- Neural networks
- Activation functions
- Biological neurons
- Space warping
- Universal approximation
- Convergence of neural networks
Backpropagation
Problem: How to compute the gradient of the loss function with respect to the weights?
(Bad) Idea: Derive ∂W∂L by hand
See what I have done Derive gradient by hand
Computational graphs
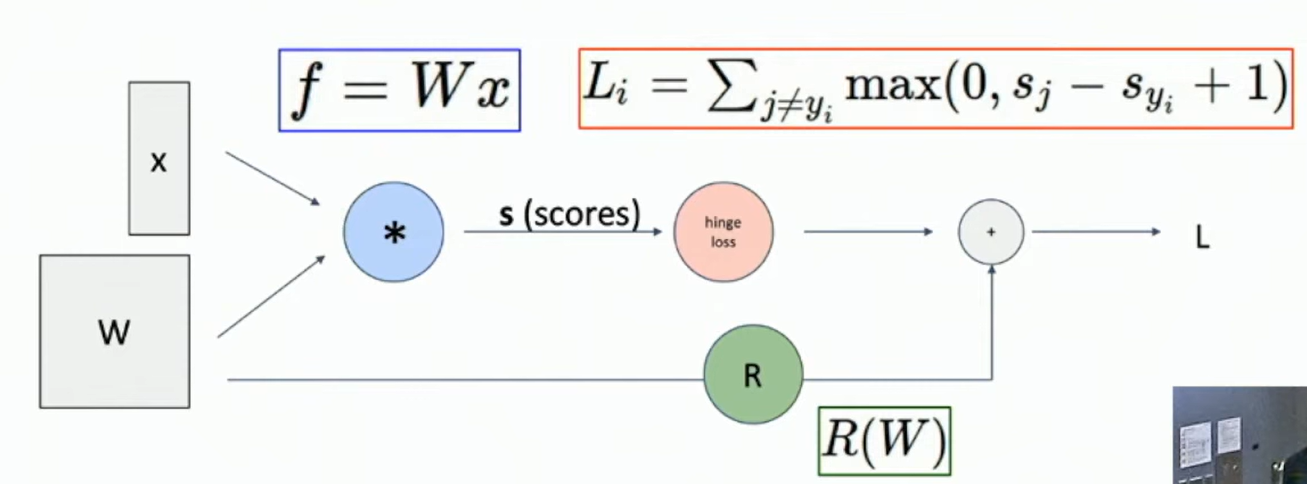
Fig: computational graph of a SVM
This is critical for large neural networks, e.g. AlexNet or Recurrent Neural Turing Machine...
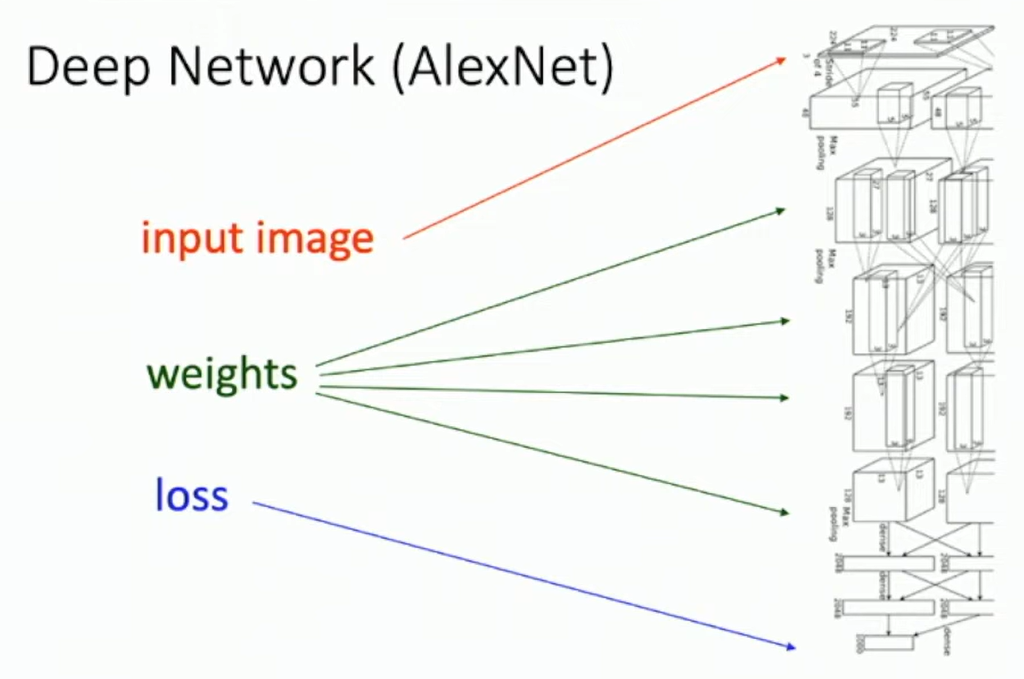
Fig: AlexNet
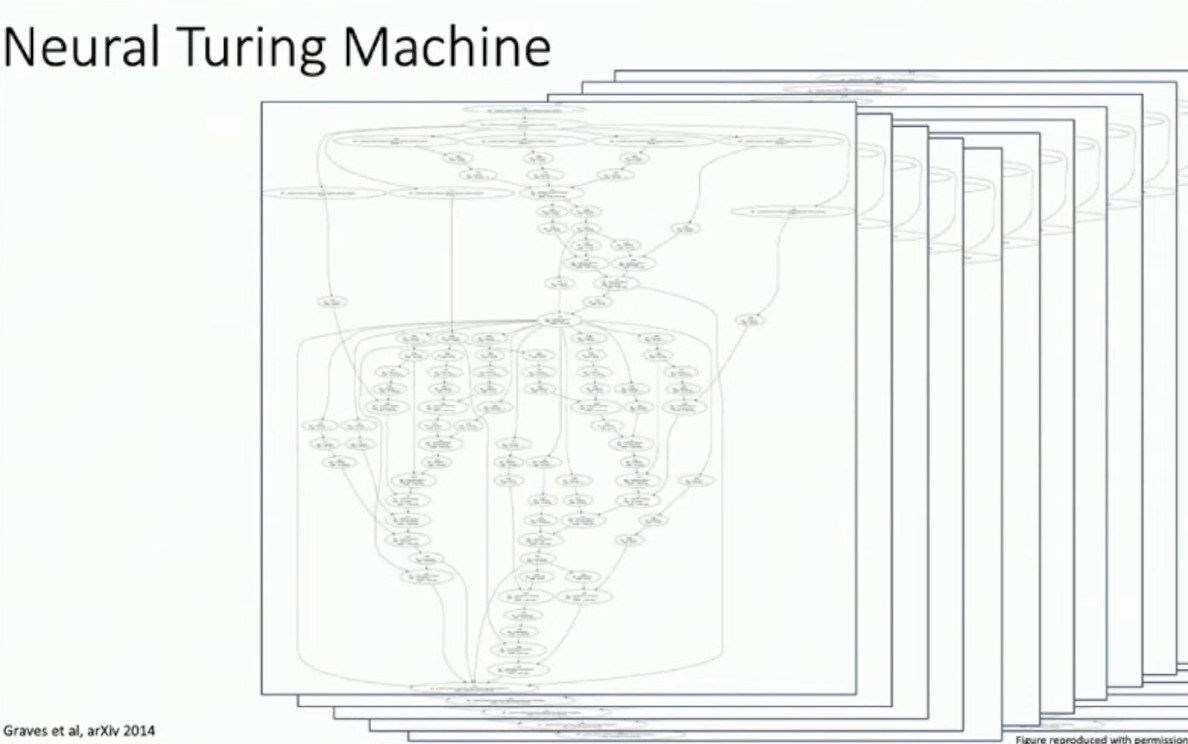
Fig: Recurrent Neural Turing Machine
Examples
Example 1
f(x,y,z)=(x+y)zx=−2,y=5,z=−4
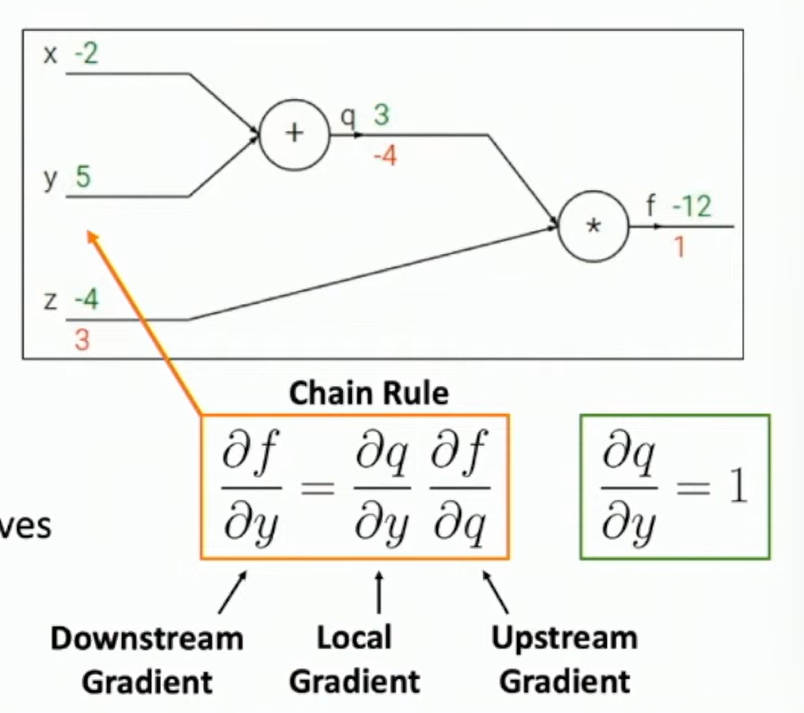
Fig: Backpropagation example 1
- Forward pass: Compute outputs q=x+y and f=qz
- Backward pass: Compute gradients ∂q∂f and ∂z∂f
∂f∂f=1∂q∂f=z∂z∂f=q
∂x∂f=∂q∂f∂x∂q=z⋅1=−4
∂y∂f=∂q∂f∂y∂q=z⋅1=−4
We can compute local gradient of one node and the chain rules can be implemented as modules.
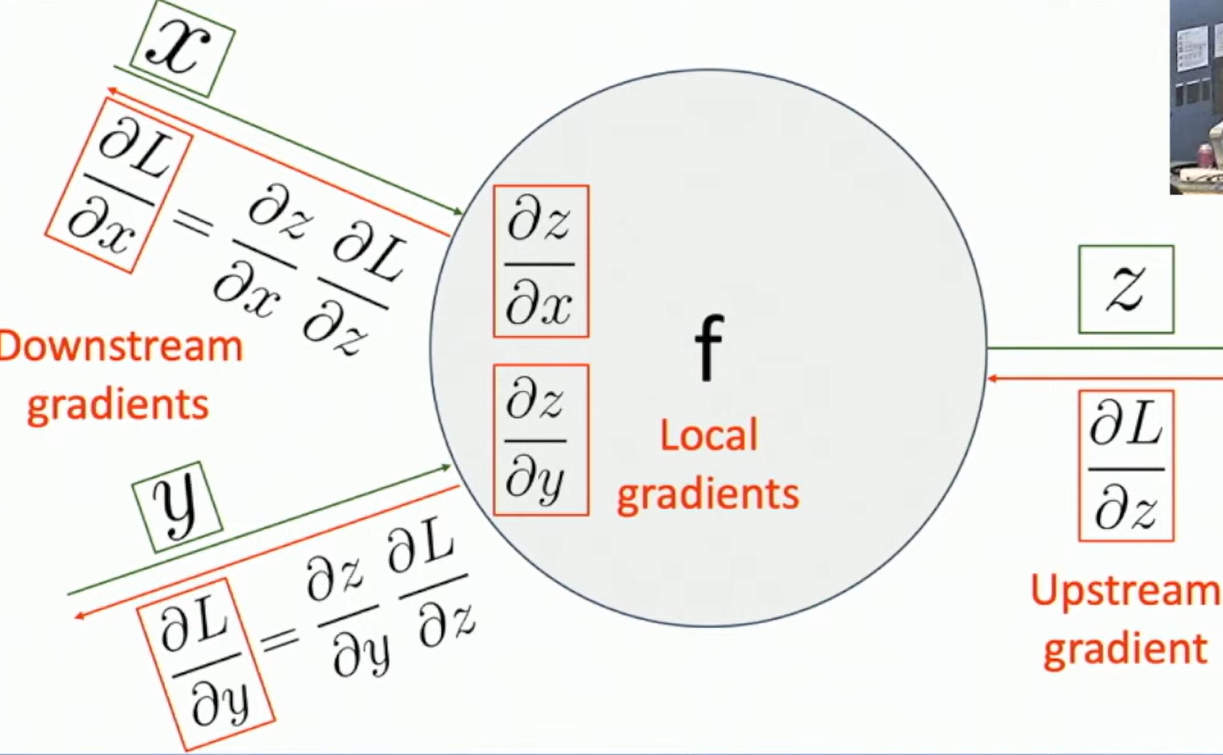
Fig: local gradient computation
Example 2
f(x,w)=1+e−(w0x0+w1x1)1
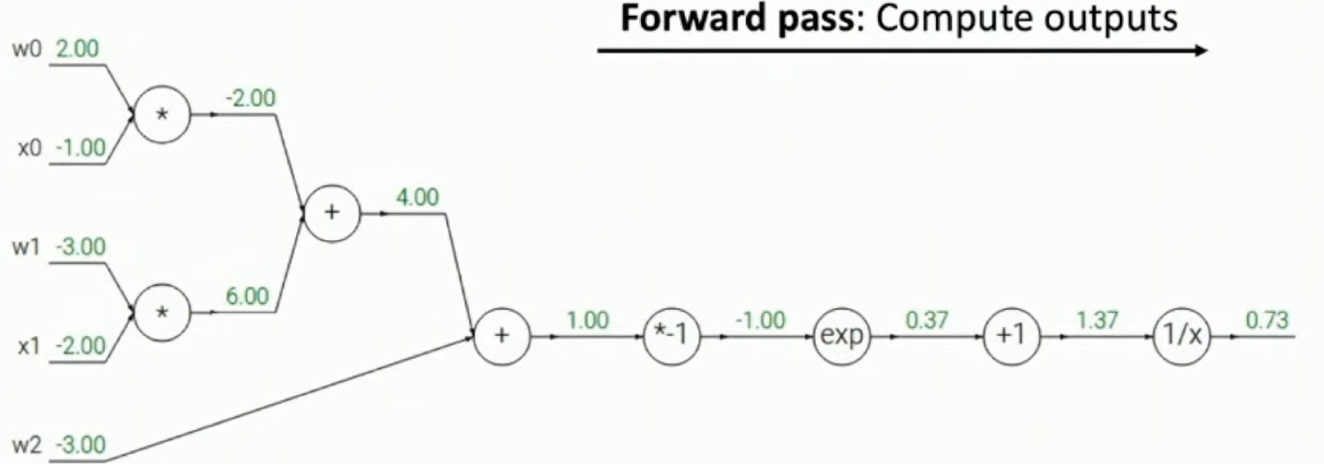
Fig: Backpropagation example 2
We can also choose more primitive functions for which the gradients are easy to compute.
e.g. Sigmoid functions
σ(x)=1+e−x1dxdσ=σ(x)(1−σ(x))
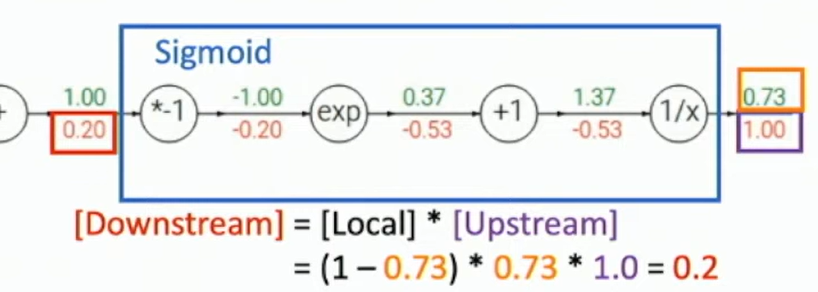
Fig: Sigmoid function
Pattern in gradient Flow
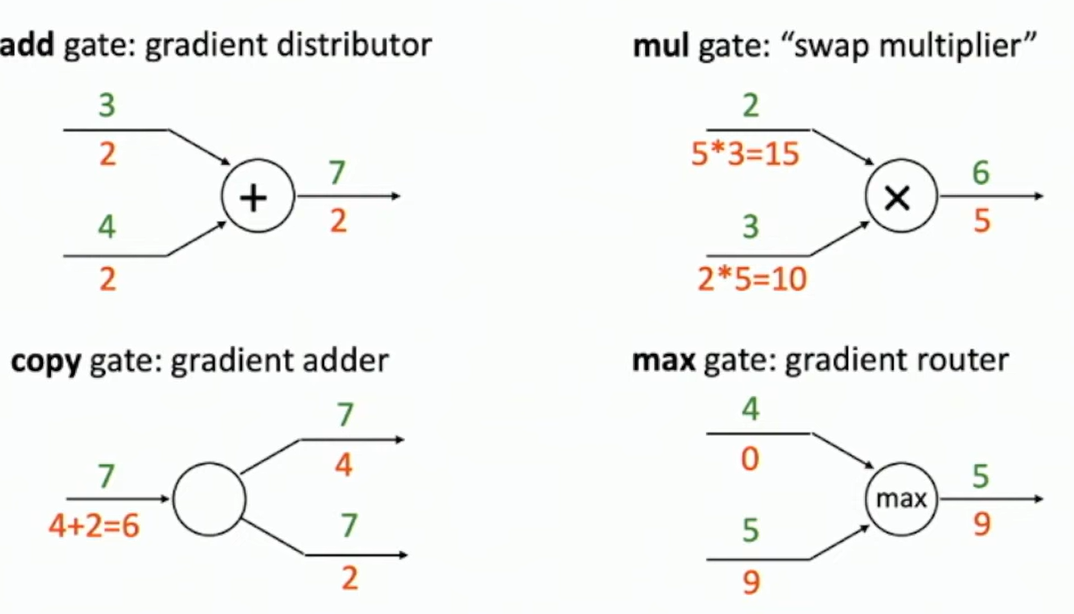
Fig: Patterns in Gradient flow
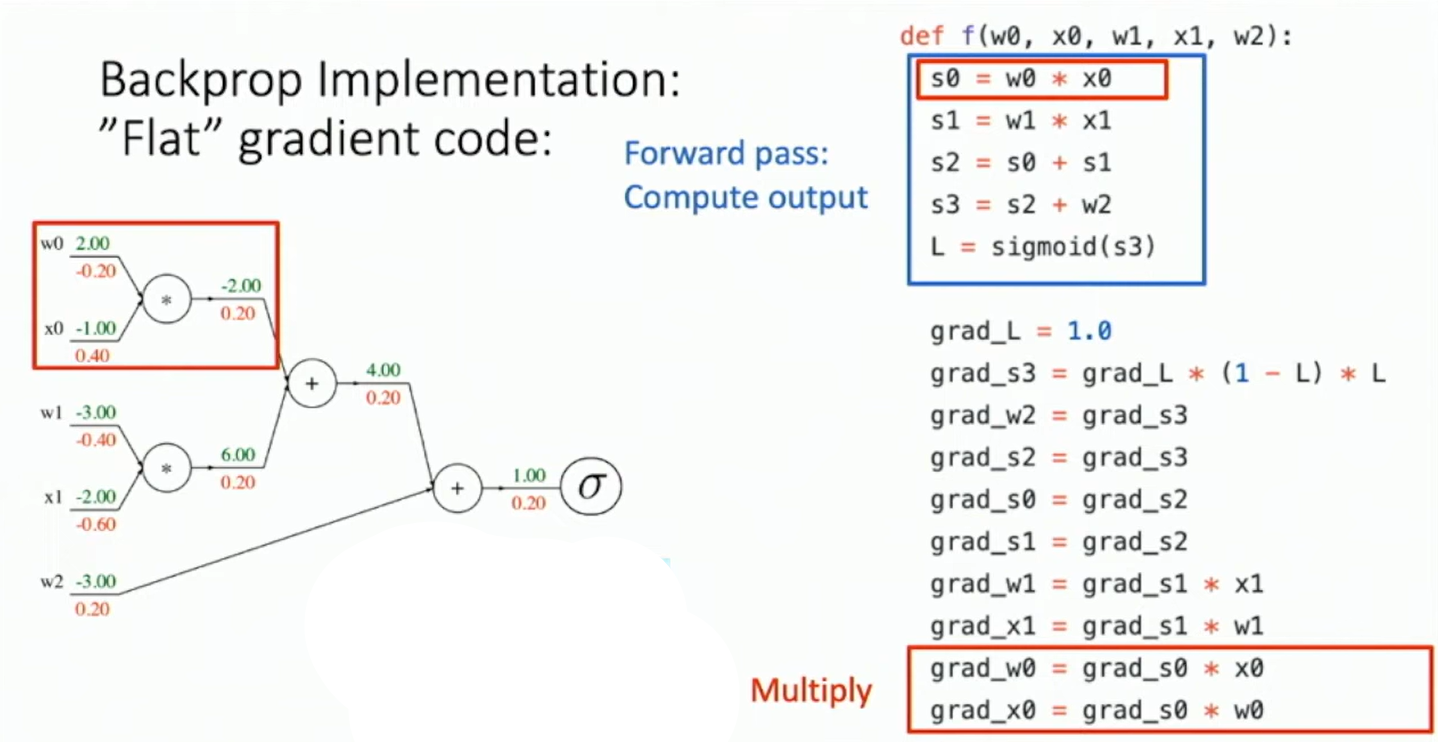
Fig: Backprop Implementation
Modular Implementation
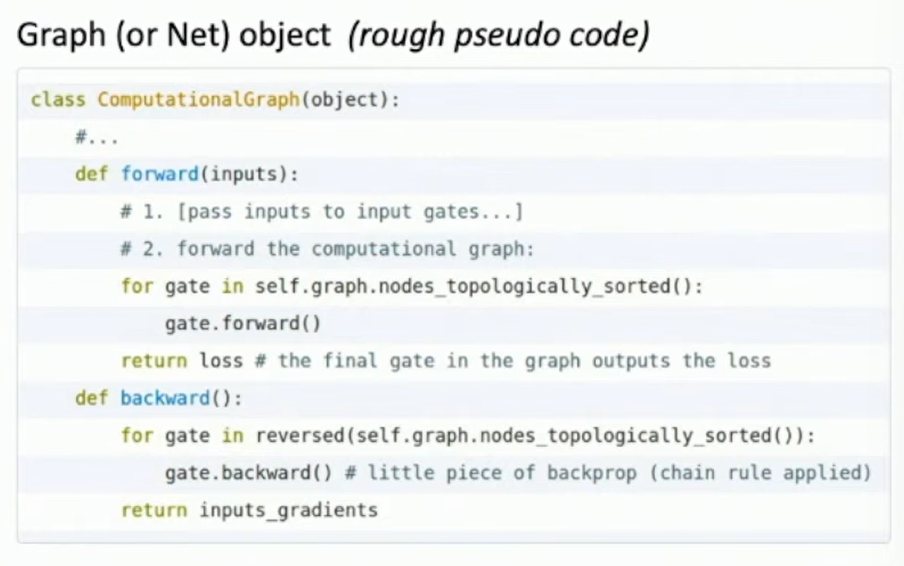
Fig: Modular Implementation
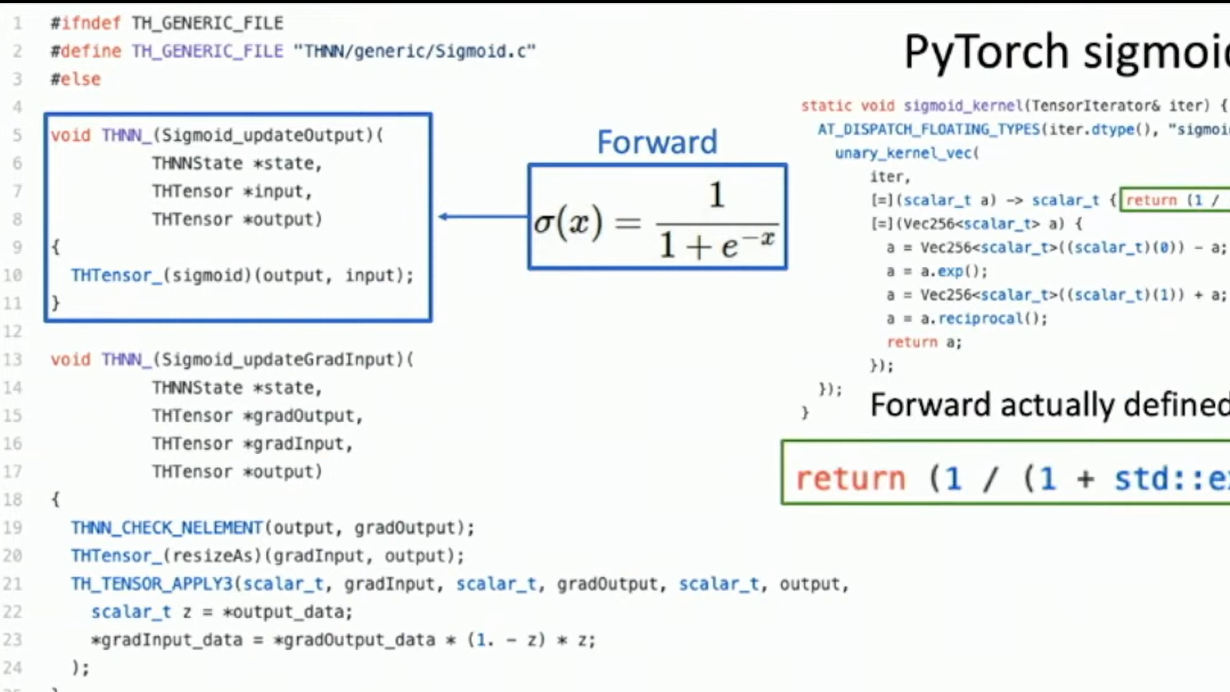
Fig: Backpropagation of Sigmoid function
Backpropagation for vector-based functions
In this course, the jacobian matrix is the transpose of that in mathematics...
∂x∂f=∂x1∂f1⋮∂xn∂f1⋯⋱⋯∂x1∂fm⋮∂xn∂fm∈Rn×m
Local gradient calculation:
Let f be a vector-valued function f:Rn→Rm, and y=f(x). Suppose we have calculated ∂y∂l∈Rm, then
grad_x∂x∂l=local grad module∂g∂f(x)⋅grad_y∂y∂l(y)
Again we have omitted transposing ∂y∂l.
In practice, we never actually compute the jacobian matrix, but instead compute the product of the jacobian and a vector.
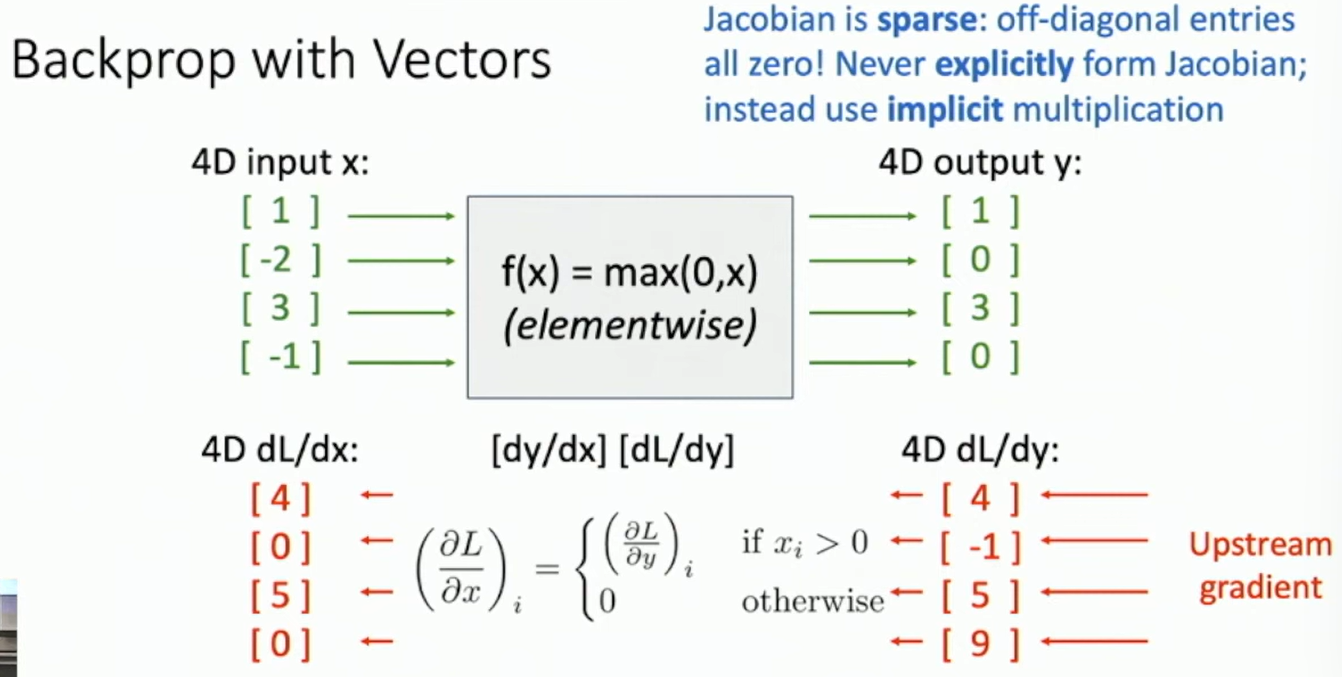
Fig: Backpropagation for vectors
Backpropagation for Matrices (or Tensors)
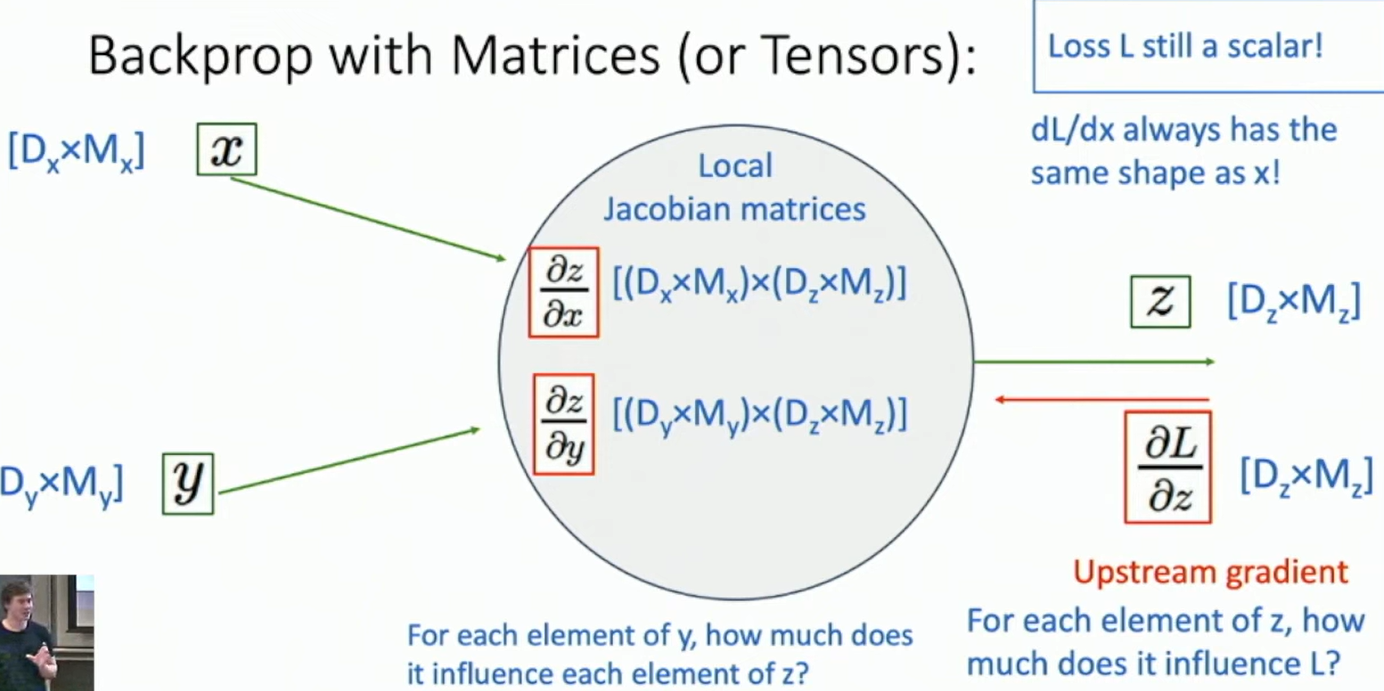
Fig: Backpropagation with Matrices
How to generalize the matrix product?
We can use the Einstein summation convention to generalize the matrix product.
Let X∈Rm×n and a matrix function Y=f:Rm×n→Rp×q. Suppose we have calculated ∂Y∂l∈Rp×q, then
∂X∂l=∂X∂f(X)⋅∂Y∂l
where ∂X∂f(X) is a tensor of shape (m,n,p,q).
With the Einstein summation convention, we can write this as
∂X∂lij=∂Xij∂fkl∂Ykl∂l
The trick here is to find a quick way to carry out the computation implicitly without actually constructing the jacobian tensor.
Reverse-Mode Automatic Differentiation
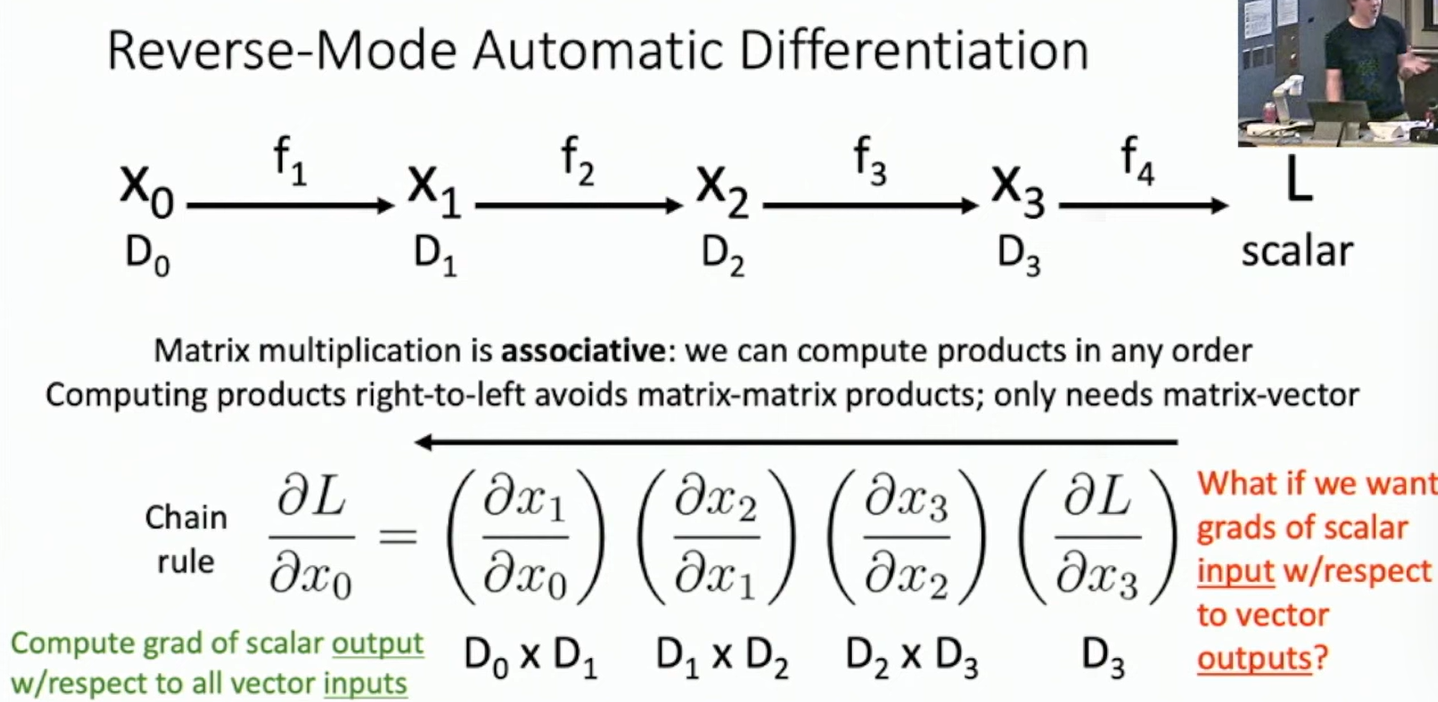
Fig: Reverse-Mode Automatic Differentiation
Forward-Mode Automatic Differentiation
This is used when the input is a scalar.
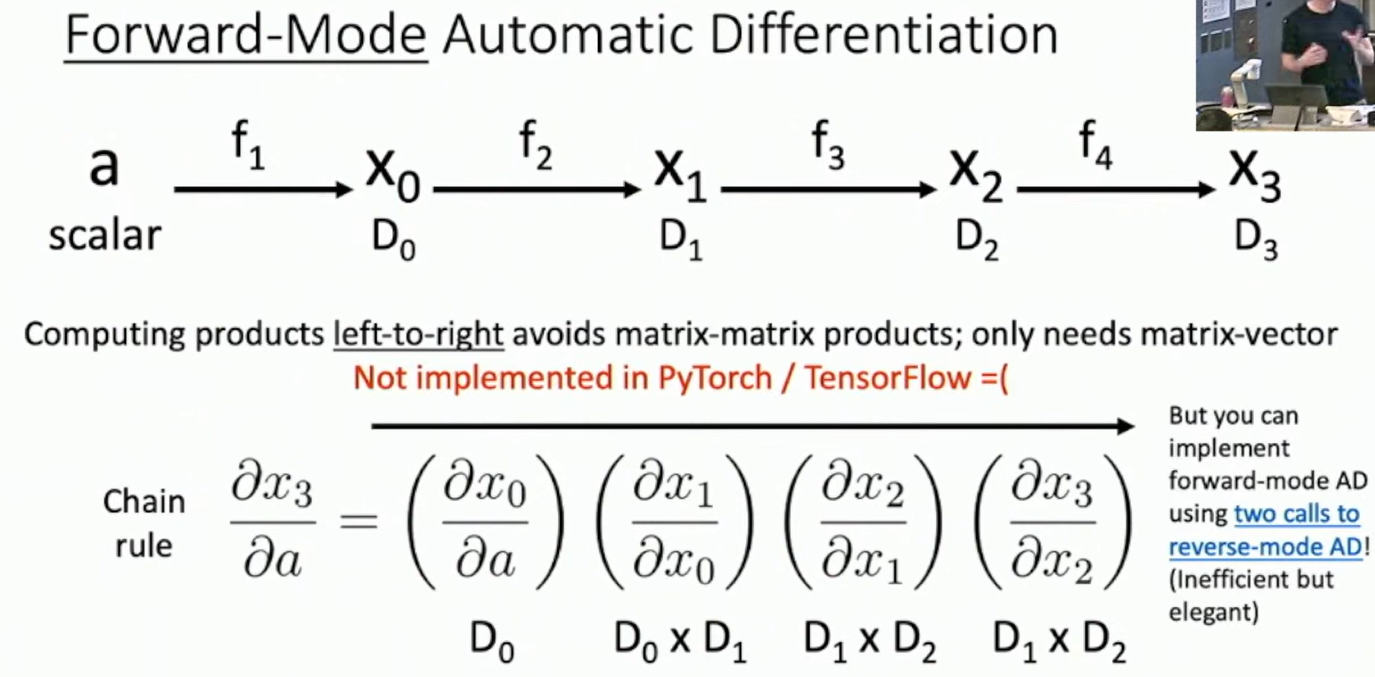
Fig: Backpropagation with Matrices
Backprop: Higher-Order Derivatives
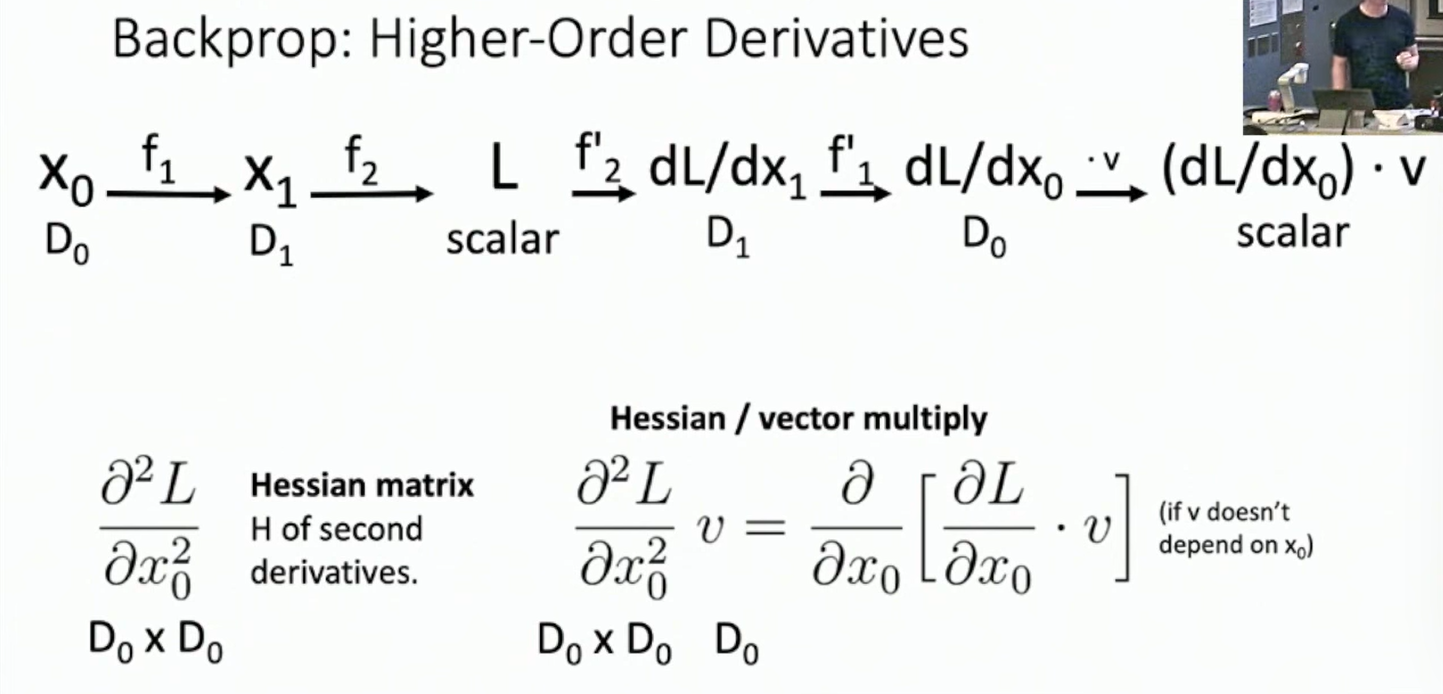
Fig: Higher-Order Derivatives
If we want to calculate the Hessian/vector product, we can construct this by hand.
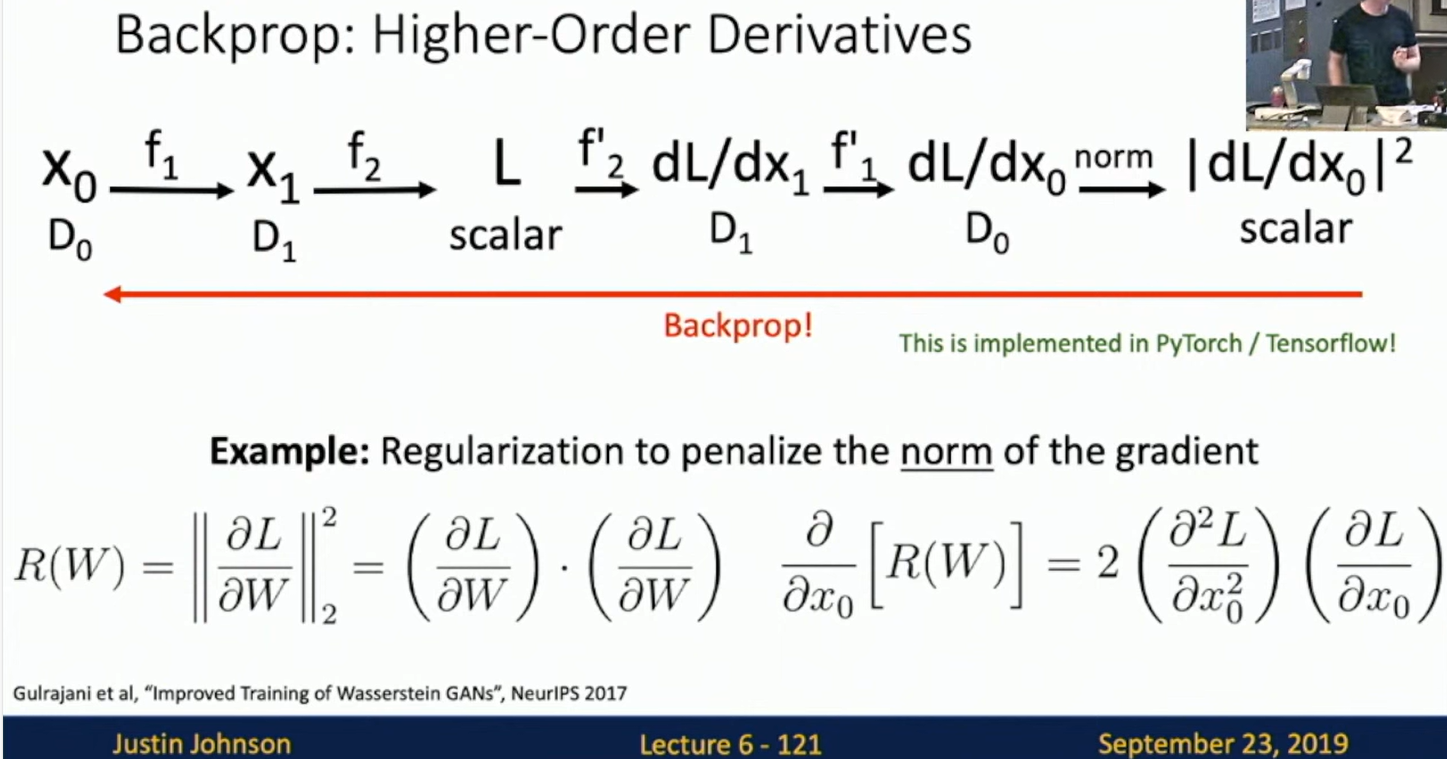
Fig: Application of Higher-Order Derivatives as a penalization
Summary of lecture 6
- Backpropagation
- Computational graphs
- Examples of backpropagation
- Gradient flow patterns
- Modular implementation
- Backpropagation for vector-based functions
- Backpropagation for matrices (or tensors)
- Reverse-mode automatic differentiation
- Forward-mode automatic differentiation
- Higher-order derivatives
Notice on Usage and Attribution
This note is based on the University of Michigan's publicly available course EECS 498.008 / 598.008 and is intended solely for personal learning and academic discussion, with no commercial use.
- Original Course Resources: Please refer to the official University of Michigan website for complete and accurate course materials.
- Third-Party Open Access Content: This note may reference Open Access (OA) papers or resources cited within the course materials. These materials are used under their original Open Access licenses (e.g., CC BY, CC BY-SA).
- Proper Attribution: Every referenced OA resource is appropriately cited, including the author, publication title, source link, and license type.
- Copyright Notice: All rights to third-party content remain with their respective authors or publishers.
- Content Removal: If you believe any content infringes on your copyright, please contact me, and I will promptly remove the content in question.
Thanks to the University of Michigan and the contributors to the course for their openness and dedication to accessible education.